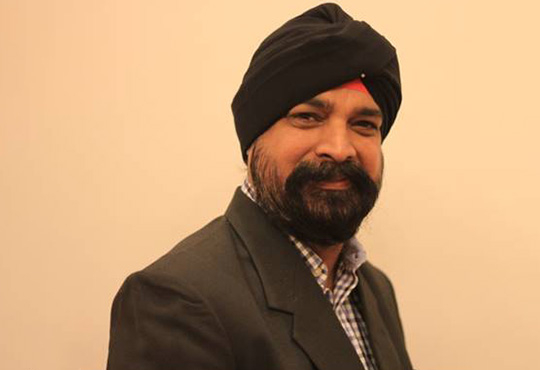
Fast data and big data - Two sides of the same coin
Souvik Bose, CIO, Delgence Technologies Pvt Ltd
So we are in 2016 and Big data has been quite a buzzword in the IT world for past couple of years now. But what's the next big thing in Big data itself and how the landscape of how we process data is changing?
As we always know that necessity is the mother of all invention, and living in 2016 has given birth to the necessity of fast data. Today we all generate huge volume of data with huge velocity but don't have much time in our hands to process them using traditional big data technologies like batch processing and wait for hours to get meaningful analysis based on that data. Today businesses need real-time analysis of data including external data like social media data and drive their business in right direction based on the meaningful insights provided by the real- time analytics from the data.
Fast Data: The fire-hose of data
To understand how fast actually the fast data is, let’s focus on some of the statistics. By the time you finish reading this article, 2 million post has already been shared by the facebook users worldwide, about half a million tweets has came into existence now and 1 million photos have been uploaded in instagram. All this happened when you are reading about fast data here. So it is very easy to understand the pace at which data is generated in today's world and it is no longer possible to run meaningful ad-hoc analysis of fire-hose data using traditional data mining techniques.
Technology behind fast-data: the other side of today's big data
In 2013, the definition of big-data was primarily revolved around 3 V's (Velocity, Volume and Variety). But later more V's like Veracity were added to extend this definition. Apache Hadoop as we know the primary technology behind Bigdata revolution was based on the idea of map-reduce and batch processing. In those times real-time data processing was not possible at all. However with changing business needs Map-Reduce 2.0 was introduced in no time termed as Apache Yarn. However with the fast changing need emerging and to meet up growing demands of data processing, in memory technologies of data processing projects like Apache Spark and Twitter Storm became much more prevalent. This is not only changing the scenario of open-source Apache projects of the big data ecosystem, but big proprietary giants like SAP came up today with products like SAP Hana which is an in-memory platform to process big data. The focus shift from file batch processing to in-memory computation in today's big data world can be seen everywhere. Just a year back high level layer above map-reduce programs such as Apache Hive took a considerable amount of time to return meaningful data warehousing result, but today with the help of Cloudera Impala and Hive streaming it is possible to achieve near real-time analysis done on fairly complex set of data. Also cloud computing platform like Amazon AWS are also changing and bringing in more and more memory optimized instances and technologies like Elastic Map Reduce to make things compatible with fast data. So in other words it can safely be said as today's big data is just the other side of the new buzzwords in the industry fast data.
Business achievements of fast data
In order to identify a customer and serve the best products for them retail giants like Walmart are using big data (fast-data techniques) to offer their customers in real time. Hospitality industry like Denihan group in Manhattan is identifying the customers and serving them with the best greetings and room offer even before they enter through the main gate of the hotel. Airlines are able to predict engine failure on aircraft flying over 35K feet even before their occurrence and save millions of dollars and most importantly human lives. Small scale entrepreneurs are able to monitor and adjust real time site traffic and their behavior my analyzing log data fast and optimizing on their paid ads revenue.
Ideal technology deployment needed
Deploying a big data cluster on the updated cloud computing instance like Amazon AWS EC2 with high memory optimized clusters like m3.large or m3.xlarge along with latest distribution of hadoop ecosystem from Cloudera or Hortonworks will make us technically much more equipped to handle fast data. Additionally deployments like Apache Spark, Twitter Storm with NoSQL like Cassandra and full scale systems like SAP Hana based on the business need required will solve the purpose of analyzing fast-data in most cases.
But as with these great technologies, people obviously need skilled and updated technology partners and data scientists who are always up to date on latest technology of the big data sphere and able to select and suggest the best deployment. Because like we see here today fast data and big data are really two sides of the same coin, and fast is becoming faster everyday.
CIO Viewpoint
Aligning IT Roadmap with Business Objectives: A...
By Subhash singh Punjabi, CISO & Head Enterprise Architecture, Deepak Fertilisers & Petrochemicals Corporation Ltd
Empowering Women: Shaping the Future of Industry
By CIOTechOutlook Team
Scaling AI: Finding the right Biztech...
By Sujatha Gopal, CTO - Communications, Media & Information Services (CMI), Tata Consultancy services
CXO Insights
Multiplying Opportunities In Big Data Analytics
By Diwakar Chittora, Founder & CEO, Intellipaat
Incorporating Blockchain Capabilities into...
By Tamal Chowdhury, SVP, Artificial Intelligence, Course5i
Digital Transformation & Working Remotely Is...